At Mind Moves, we’re committed to helping federal agencies unlock the potential of Artificial Intelligence (AI) to drive meaningful, responsible business transformation. In pursuit of this mission, we lead initiatives that prioritize practical design principles while envisioning an inclusive and equitable AI future.
We’re thrilled to share insights from a recent Generative AI (GenAI) project with the National Library of Medicine (NLM) at the National Institutes of Health (NIH). This high-effort initiative tackled complex challenges in the rapidly evolving AI landscape. Our team’s achievements, delivered in just three months, showcase how we help clients reach their goals with the latest in GenAI technology. Here’s what we learned, and what might help you in your own AI journey.
GenAI Minimum Viable Product (MVP) Objectives
At Mind Moves, we view GenAI development as a journey from experimentation to MVP, and eventually, to operational integration. Building upon the NLM GenAI Pilot, our goal for this phase was to develop basic Large Language Model (LLM) applications that could be tested in real-world workflows. Our technical approach included a robust backend featuring Retrieval Augmented Generation (RAG), vector databases, and model fine-tuning – all within a secure, firewalled cloud environment.
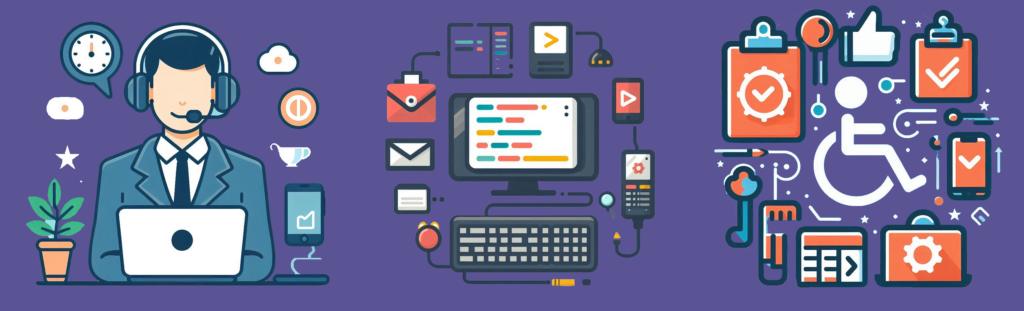
Key business objectives included:
- Enhancing customer service through GenAI-powered responses to consumer-health inquiries, improving efficiency and customer satisfaction.
- Streamlining metadata mapping with Python-coded scripts to reduce costs and accelerate data discovery.
- Improving accessibility and compliance with 508 standards by employing automatic detection and resolution programs.
The Development Process
Given the three-month window, delivering fully functional MVPs required a streamlined and agile approach. We adopted Agile methodologies, organized into sprint cycles, to navigate each stage, from setting up environments to refining model outputs. Weekly check-ins, JIRA for task management, and support from Microsoft’s Azure Office Hours were invaluable for staying on track. However, we faced some administrative delays in setting up environments and permissions, compressing our timeline and necessitating extra flexibility to meet deadlines.
To balance technical demands, we paired our project leads with machine learning engineers, enabling the project leads to focus on learning and testing GenAI methods without being overwhelmed by technical complexities.
As we moved from prototype to MVP, we aligned our processes with the NIST AI Risk Management Framework: Generative Artificial Intelligence Profile (released in April of this year). Collaboration with internal experts ensured that human oversight was maintained at key decision points.
Organizational Impact
Despite challenges, each MVP project successfully met its objectives, with some especially well-positioned for future enhancements. For example, the Customer Response project, which engaged Customer Service Representatives (CSRs) throughout the development process, helped build trust and confidence in GenAI solutions.
One of the most impactful achievements was the development of a containerized, cloud-agnostic solution, compatible with AWS, GCP, and local environments. This flexible design offers scalability and adaptability for future needs.
Our work also established foundational technical services, such as RAG pipelines and vector store creation, which will be foundational to future GenAI integration. Final demonstrations to NLM leadership showcased high Bertscores (with an F1 average of .80 out of 1), time savings, and practical utility that met expectations, further proving the effectiveness of these solutions.
Notably, one MVP led to recognition at the NIH level. The project lead received the 2024 NIH Award for Accessibility, thanks to our ChatGPT-driven tool that identified accessibility issues on NLM’s website and recommended actionable solutions, greatly improving overall accessibility.
Key Learnings and Recommendations
Reflecting on our experience with AI integration projects, we have several recommendations for similar initiatives:
- Extend Pilot Timelines: Future projects could benefit from 9–12-month cycles to accommodate complex development needs and varying team availability.
- Improve Pre-Pilot Preparation: Addressing administrative requirements, like permissions and environment setup, before launch can prevent delays.
- Adopt Flexible Timelines: Tailor timelines to align with the development needs of individual projects.
- Foster Cross-functional Collaboration: Encourage knowledge-sharing across projects and build communities of practice to drive innovation.
How Mind Moves Can Support You
Our track record of delivering “quick wins” in tightly scoped projects sets the foundation for broader, strategic development. NLM processes over 70,000 health-related queries from the public annually. Currently, responses are provided by humans, which can lead to delays and subjective variability. Leveraging AI as a research assistant could significantly enhance response throughput, reduce customer wait times, and free up CSR agents to focus on other high impact tasks. Our evaluation has shown that AI RAG pipelines generated responses that were close to 70% aligned with expert generated responses.
Our diverse team of experts works tirelessly on the edge of AI advancements, helping leaders and organizations to rethink problem-solving through safe, human-centered AI solutions. Reach out to explore how we can support you on your AI journey.